Federica Bianco’s Research Ranges From Outer Space To Human Cities
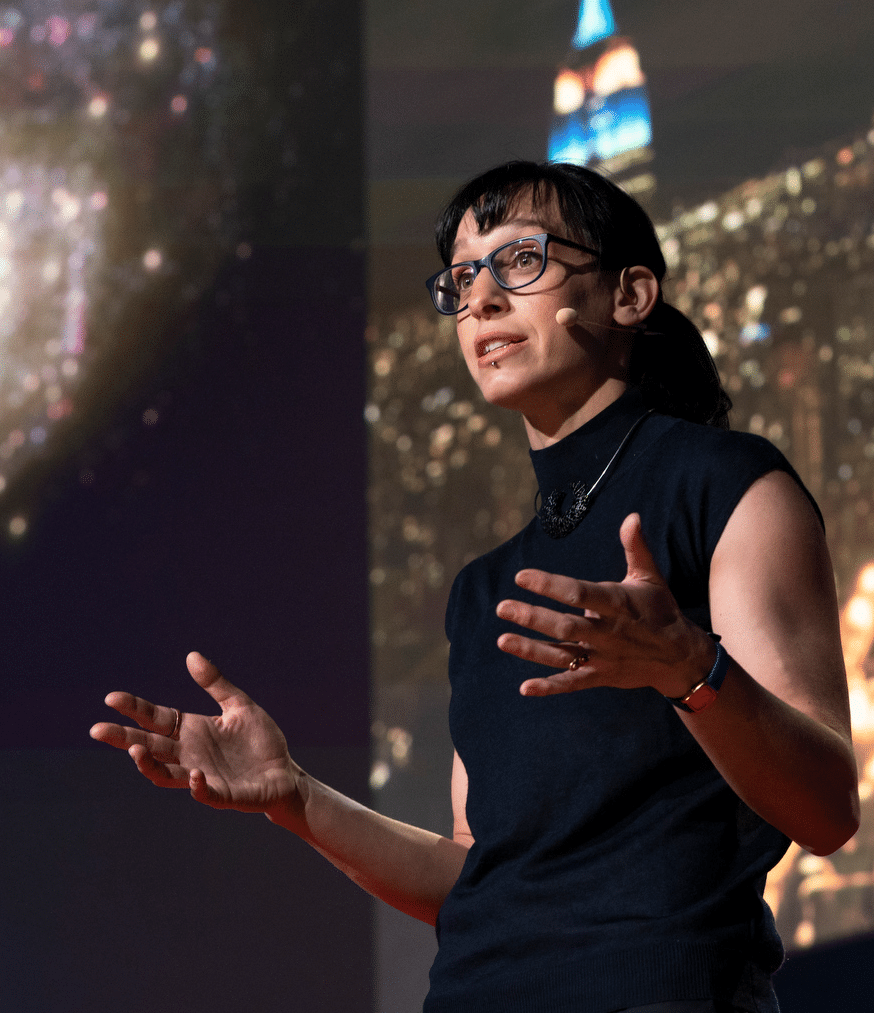
It’s hard to pin Federica Bianco down. Literally, because the University of Delaware researcher moonlights as a professional boxer named “The Mad Scientist”—but also figuratively, because she studies so many seemingly unrelated topics, from astrophysics to urban planning. She’s even dabbled in public health: During the pandemic, she led a team to develop and run a model that Delaware’s government used to predict demand for hospital beds.
The throughline? All of Bianco’s projects involve analyzing large amounts of data. Her analysis techniques often translate, regardless of the subject of the dataset. “If anything, my superpower in collaborations has become adapting methods from one discipline to another,” says Bianco.
Bianco, a native of Genova, Italy, began her discipline-hopping journey at young age. In middle school, she attended a music conservatory for classical piano. In high school, she focused on Latin and Greek. Entering university, she found physics came easily, and she eventually chose to attend graduate school at the University of Pennsylvania in the early aughts, where she performed solar system research. After earning her PhD, she stumbled into “urban science,” a field that adapts astrophysics methods to study cities, when New York University recruited her husband, also an astrophysicist, to build a facility dedicated to the field.
In recent years, Bianco added another field to her portfolio: artificial intelligence. In addition to using it in her astrophysics research, she advocates for ethical use of the technology. Bianco spoke to 1400 Degrees about her varied career.
How did you decide to become a physicist?

Photo: Ryan Lash / TED
I don’t know that I ever thought of being a professional anything. I decided I wanted to study astrophysics toward the end of high school, but in college I wasn’t focused on grad school. I do what is fun for the moment and make the best of it so long as it’s interesting. I’m not very future-oriented, and of course I have a lot of privilege to be able to live like that.
You came to the US for graduate school in 2003 and you’ve lived here ever since. Did you ever experience culture shock?
I have culture shock on a regular basis every six months or so, even twenty years after I moved. Recently, it’s been difficult to make sense of the racial reckoning in the US from my cultural background. Racism has a slightly different flavor in Europe. It’s easy to think you’re not racist because you can think of yourself as xenophobic instead—that you don’t think someone belongs because they’re a foreigner, rather than because of their skin color. But it’s the same problem, except we use different words to make ourselves comfortable.
I now get culture shock from both countries, because I live in between the two worlds. I am Italian, and I am American. I identify as both, but I identify as neither, and honestly I feel like nobody thinks of me as either. I’m just “other.” I think some of this is reflected in the science I do. It would be more comfortable to just do the science that I’m an expert in, but I’m drawn to learning and adapting.
What compels you to work in so many disciplines?
I enjoy the initial stages of a project most because that’s when you learn the most. I get distracted once I improve at something and have to start focusing on the finer details. That’s why I swap projects quite often. I’m also less motivated to study the details of a physical phenomenon. Some people do data analysis to understand how stars explode. I care less about how they explode and more about the methodological aspects themselves. I’m more fascinated in the path to answer some question.
You’ve applied techniques from astronomy to study energy usage in cities. Can you give an example?
Since grad school, I’ve studied occultations, which occur when an object in space passes in front of a background star to block some of that star’s light. We look for objects so small that even powerful telescopes can’t detect them directly, but we indirectly detect them as they pass in front of the star. But they pass quickly, making the signal short-lived and complex. Consequently, our projects require tons of data and special statistical methods.
My team and I adapted these methods to study cities. Instead of cameras pointing to outer space, we photographed New York City from hills and tall buildings. Similar to the occultations, we studied changes in light on short timescales. We developed a now-patented method for measuring high-frequency changes in electrical lights. When imaging incandescent light bulbs in the US, you can capture fluctuations in the light at 120 times a second due to each cycle of alternating current. These oscillations are synchronous for lights connected to the same transformer. So from these oscillations, we could figure out the structure of the grid, which is important information for making city plans for recovering from outages, for example. Energy companies typically keep the grid structure secret.
You also do AI research. What big questions are you interested in?
I think a lot about how we embed bias in AI. This bias already has social repercussions—law enforcement agencies are already using AI models to predict whether a convicted person will commit a crime again. These models make predictions on the basis of biased historical data, as law enforcement has disproportionately targeted people of color.
I’m interested in understanding bias in the models themselves, as opposed to bias in training data, which the field tends to focus on. People often assume the bias comes just from the data, while the model is objective. But I strongly disagree. We embed bias in our models as we build them. The big question is, how do we prevent this?
How does bias enter a model?
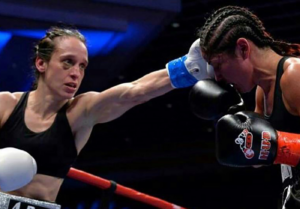
Credit: CSB Photography
A model learns to perform a task by optimizing a mathematical function known as a loss function. The name refers to examples where the model learns to minimize losses, as in a business. But loss functions train a model in all sorts of tasks, like image generation or classification.
The loss function describes the model’s goal, and it reflects the model designer’s beliefs. For example, say we believe there are seven classes of galaxies. We would then build the model to reproduce that taxonomy through our choice of loss function. But in reality, we don’t know that a taxonomy exists at all. In fact, it’s more likely that these objects occur in a continuum rather than in discrete categories. But we ask models to reproduce human decisions that are convenient but not necessarily well-founded.
How would this apply to a model that predicts whether someone will re-commit a crime?
You have to think about what you want your loss function to optimize. If my goal was to make sure that no innocent person is ever found wrongfully guilty, I’ll choose a different loss function compared to if I wanted to maximize public safety and prevent people from committing any crimes.
What are you most concerned about with respect to AI?
My main concern is that it will echo the worst aspects of our thinking. People are explicitly racist and sexist on the Internet, where a lot of training data comes from, so the models will learn and propagate this language. Because of that, I’m worried that AI will harm vulnerable populations preferentially.
These models learn from reality, but reality might not be what we want them to emulate. We probably want them to emulate an idealized world so that they can push us toward that. But the problem is, who gets to decide what the idealized world is?
How does your physics background inform this work?
Physicists can definitely bring a specific perspective to AI. We’re often more sensitized to understanding the peculiarities of real data, such as noise and systematics, than computer scientists who tend to work with benchmark datasets.
What makes regulating AI models different from regulating other technologies?
You can’t test AI models for safety using the same approaches as many other technologies. If you’re building a car, you can make the car safer by testing it in a restricted environment. But testing models in a controlled environment doesn’t ensure safety in the same way. Companies do have a diverse set of people beta-test the model, and they pay people to ask the models questions and make the models safer by not allowing it to use racist words or talk about supercharged topics based on those interactions. But models make their impact when they enter society.
Older technologies also took much longer to become as widespread. Electricity took decades to propagate worldwide. But technologists have released AI at large right away. In fact, Sam Altman gave an interview where he said they released GPT-3 when it sounded eloquent and coherent, but they knew the content wasn’t accurate. That was mind-blowing to me.
In an ideal world, how would you have rolled out this technology?
I don’t know that there is a right way. But I want people who have education in AI in decision-making positions. AI develops rapidly, and policymakers have to catch up. But by the time they try to catch up, that technology has been superseded, so they regulate something that has become irrelevant, and the next thing is already possibly doing damage.
I also think we need to cultivate a culture of scientific ethics among physicists and data scientists. I actually pitched a fit at my university and demanded that students getting a master’s in data science needed to take a mandatory ethics of AI class. My friend Tom Powers who is a philosopher, now teaches that class. You can’t send people in the world to work for AI companies not having taught them about the potential impact.
Sophia Chen is a science writer who covers physics, space, and anything involving numbers. Find more of her work at sophurky.com.
Want to recommend a physicist for us to profile? Write to info@1400degrees.org. All interviews are edited for brevity and clarity.